TCR repertoires from COVID-19 patients and healthy donors reveal trends in CDR3 gene usage and diversity
To determine if there were any global patterns that distinguish the immune repertoires of COVID-19 patients, we systematically compiled and analyzed TCR-seq samples (total n = 2130) from COVID-19 patients and healthy donors (Fig. 1A, Supplementary Dataset S1). TCR repertoire datasets were obtained from studies by Adaptive Biotechnologies (AB, n = 1574), ISB-Swedish COVID-19 Biobanking Unit (ISB-S, n = 266), PLA General Hospital (PLAGH, n = 20), and Wuhan Hankou Hospital (WHH, n = 15), and then uniformly processed for downstream analysis (see Methods). The individual datasets underwent separate but standardized processing using identical bioinformatic pipelines, as opposed to combining the data into one pooled set prior to analysis. All comparisons between COVID-19 and healthy donor controls were made within the individual dataset, and any reference to the findings in multiple datasets in the manuscript refers to the consensus findings of within-dataset comparisons. The analyses for the ISB-S dataset were further stratified by cell type (CD4 vs CD8), separated into ISB-S CD4 and ISB-S CD8 datasets, both of which contain TCR sequences of healthy donors and COVID-19 patients. Clonality analyses revealed that COVID-19 patient samples from the ISB-S CD4, ISB-S CD8, and WHH datasets had fewer total unique clonotypes compared to healthy donor controls in these respective datasets, demonstrating the consistency of this observation in multiple sources of TCR data (Fig. S1A). Moreover, repertoire diversity metrics, including Chao1 estimators (a measure of species richness), Gini-Simpson indices (probability of interspecific encounter), and inverse Simpson indices, were decreased for COVID-19 samples compared to healthy donor samples, notably for the AB, ISB-S CD4, and ISB-S CD8 datasets (Fig. 1B, C, S1B). The decrease in clonal diversity measures is consistent with the increase in the relative abundance of the top clonotypes in the repertoire space for COVID-19 samples (Fig. 1F, S3D, Supplementary Dataset S2), which suggests the expansion of a small number of functional clones after antigen exposure. These results together reveal global shifts in immune repertoire clonality and diversity in patients with COVID-19 compared to healthy donors. However, one intrinsic limitation to assessing TCR diversity is the fact that the dataset’s size influences the diversity measurements, as the distribution of TCR frequencies is not linearly scalable. The higher variance in clonal diversity of COVID patients observed in the AB dataset (Fig. 1B, C, Supplementary Dataset S4) illustrates this limitation. The larger spread in clonal diversity in the COVID group in the AB dataset reflects a greater heterogeneity in T cell repertoire changes upon COVID infection compared to other datasets, but the general directionality of this change is a decrease in diversity.
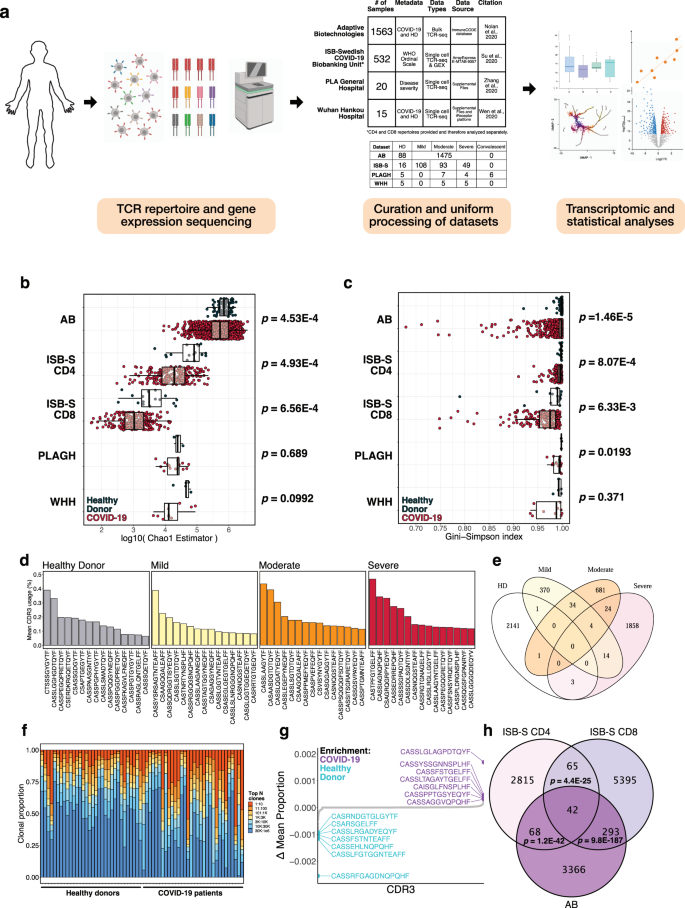
a Schematic detailing curation and analysis of TCR repertoire datasets from healthy donors and COVID-19 patients. Sequencing data was obtained from Adaptive Biotechnologies (AB, n = 1574), ISB-Swedish COVID-19 Biobanking Unit (ISB-S, n = 266, CD4 and CD8 repertoires), PLA General Hospital (PLAGH, n = 20), and Wuhan Hankou Hospital (WHH, n = 15). Created with BioRender.com. b Boxplot of Chao1 indices for COVID-19 patients and healthy donors for each repertoire dataset. P-values were obtained using the two-sided Wilcoxon rank sum test. c Boxplot of Gini-Simpson indices for COVID-19 patients and healthy donors for each repertoire dataset. P-values were obtained using the two-sided Wilcoxon rank sum test. d Bar plots showing the top 15 mean CDR3 usages for patients in the ISB-S CD4 dataset grouped by disease severity (healthy donor = 16, mild = 108, moderate = 93, severe = 49). e Venn diagram showing overlap of top mean CDR3 usages (proportion threshold = 0.0001) for patients in the ISB-S CD4 dataset grouped by disease severity. The CDR3 sequences enriched in the COVID patients have overlap among mild, moderate, and severe patients, while minimal overlap is observed between healthy donors and COVID-19 patients. f Bar plot depicting relative abundance for groups of top clonotypes for a random sample of repertoires (healthy donors = 32, COVID-19 = 32) from AB dataset, with relative overrepresentation of specific clonotypes in COVID-19 patients. g Dotted waterfall plot of CDR3 gene usage differentials between COVID-19 patients and healthy donors (delta mean proportion) in AB dataset. Purple dots are CDR3 sequences enriched in COVID-19; light blue dots are CDR3 sequences enriched in healthy donors; gray dots are all other CDR3 sequences. h Venn diagram showing overlap of COVID-19 enriched CDR3 sequences for patients in the ISB-S CD4, ISB-S CD8, and AB datasets (thresholds 0.0001 for ISB-S samples, 0.00001 for AB samples). P-values for overlap significance calculated using hypergeometric test.
To determine the specific gene usage preferences and dynamics in COVID-19 patients, we performed comparative analyses of the V(D)J gene and complementarity-determining region 3 (CDR3) gene usage for the AB and ISB-S datasets. While we observed selective V and J gene usage differences between the TCR samples of COVID-19 patients and healthy donors from the AB dataset (Fig. S1D, E, Supplementary Dataset S5, S6), fewer differences in these gene usages were observed from the ISB-S datasets (Fig. S2A–D). Moreover, there were no differences in clonotype frequencies by CDR3 length across the datasets (Fig. S1C, Supplementary Dataset S3). By comparison, the top CDR3 sequences of healthy donors, mild COVID patients, moderate COVID patients, and severe COVID TCR patients’ repertoires were different within the AB dataset, as well as within the ISB-S datasets (Fig. 1D, S3A, B). To identify COVID-19-associated CDR3 sequences that are conserved across disease conditions and datasets, we performed a series of set analyses using sequences above a proportion threshold (0.0001 for ISB-S samples, 0.00001 for AB samples, where the different proportion thresholds were applied to account for the AB dataset’s relative size compared to the ISB-S dataset) for each condition. We found that the CDR3 sequences enriched in COVID patients had overlap among mild, moderate, and severe patients, while there was almost no overlap in CDR3 enrichment between healthy donors and COVID-19 patients in both CD4 T cell (Fig. 1E) and CD8 T cell datasets (Fig. S3B). Moreover, we observe 42 conserved CDR3 sequences when comparing the union set of disease-associated CDR3 sequences for ISB-S CD4 samples, the union set of disease-associated CDR3 sequences for ISB-S CD8 samples, and COVID-19 CDR3 sequences for the AB samples (Fig. 1H). In order to determine enriched CDR3 sequences for each dataset and disease conditions, we plotted the difference in mean CDR3 proportions between samples of interest and healthy donors (Fig. 1G, S3E–J). Although the identified sequences may not be definitively specific, we provide here a set of systematically processed COVID-19-associated convergent and enriched CDR3 gene usages.
K-mer and motif analyses reveal patterns associated with disease conditions
Sequence convergence of immune repertoires can also occur at the level of motifs, or sequence substrings, in addition to that of clones. One approach to decomposing CDR3 sequences into motifs is by using overlapping k-mers, or amino acid sequences of length k, which provide a functional representation of the repertoires with increased compatibility for statistical analyses and machine learning methods24. We created 3-mer, 4-mer, 5-mer, and 6-mer frequency matrix representations of ISB-S CD4 and ISB-S CD8 datasets and performed principal components analysis (PCA) to see whether samples cluster by disease severity (Fig. 2A, C, S4A–F, Supplementary Dataset S7). We found that while the majority of samples clustered together, a number of mild and moderate samples were separated from the central cluster across all analysis permutations, while severe samples are generally associated with the central cluster of samples including healthy donors. Because there are general rules that define many CDR3 amino acid sequences, such as the CASS motif commonly found at the beginning of many CDR3 sequences, it was expected that most of the data would cluster together regardless of COVID infection status. The homogenous signal of shared CDR3 characteristics was likely to dominate the heterogenous CDR3 k-mers that differentiate individuals’ repertoires. However, the outliers in the PCA that failed to fall into the large central cluster, which came from mild and moderate COVID samples, possibly indicated that the PCA is detecting high-variance data features that differentiate them from other CDR3 k-mers, while all severe COVID samples were found within the homogenous central cluster. Machine learning is a broadly useful tool to detect and identify these fine differences in biological signal. The outliers from mild and moderate COVID patient samples may suggest that T cell repertoires may be undergoing changes that selectively enrich certain clones that harbor specific TCR motifs, in response to COVID infection, which is being captured in the PCA plot. No such changes were detected in severe COVID patients’ TCR motifs in the PCA. These results are consistent with emerging data that patients with severe COVID-19 have substantial immune dysregulation in comparison to those with less severe disease. Studies have shown that T cell polyfunctionality is increased in patients with moderate disease but reduced in those with severe disease25, and there have been proposed models of TCR clonality whereby the response in mild disease includes detection of dominant clones while the response in severe disease do not26. Moreover, heatmaps of 3-mer abundances reveal some shared motifs between mild and moderate samples such as YNE, NEQ, EQF, and QFF for repertoires randomly sampled from the ISB-S CD4 dataset and TEA, EAF, and AFF for repertoires randomly sampled from the ISB-S CD8 dataset (Fig. 2B, D). In aggregate, these results suggest that there are sequence features that distinguish COVID-19 TCR repertoires from healthy donors to various degrees based on disease condition.
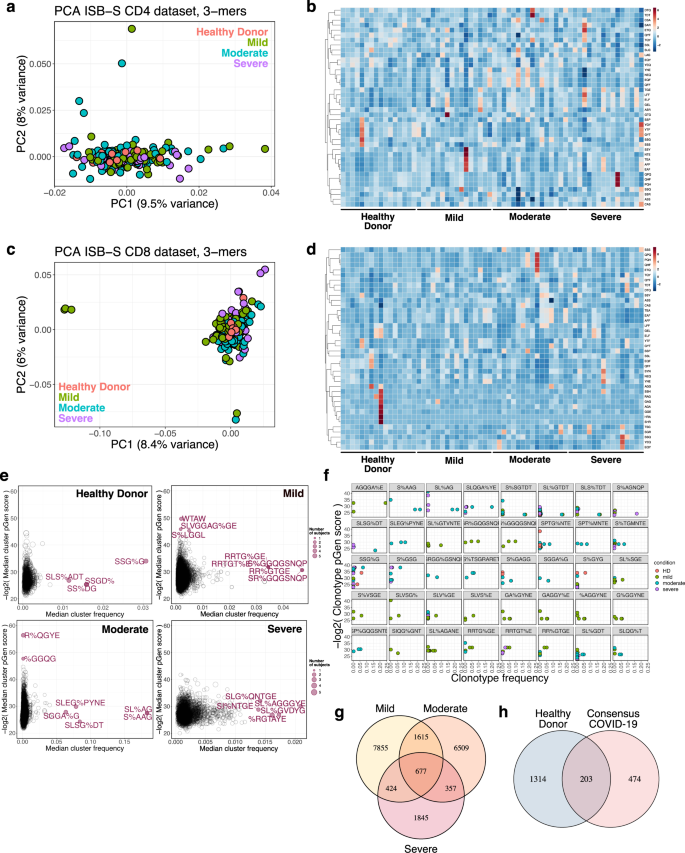
a Principal components analysis of 3-mer representations of TCR repertoires from the ISB-S CD4 dataset (n = 266). b Heatmaps of 3-mer abundances of a random sample of repertoires from the ISB-S CD4 dataset by disease condition (healthy donor = 16, mild = 16, moderate = 16, severe = 16). c Principal components analysis of 3-mer representations of TCR repertoires from the ISB-S CD8 dataset (healthy donor = 16, mild = 108, moderate = 93, severe = 49). d Heatmaps of 3-mer abundances of a random sample of repertoires from the ISB-S CD8 dataset by disease condition (healthy donor = 16, mild = 16, moderate = 16, severe = 16). e Median frequency and pGen scores of COVID-19 and healthy donor associated T cell clusters from GLIPH2 analysis of the ISB-S CD4 dataset, grouped by disease condition. f Detailed view of frequencies and pGen scores of specific clonotypes associated with high frequency T cell clusters from CD4 dataset. Clonotypes are colored by patient disease condition. g Venn diagram showing COVID-19-associated T cell clusters for patients in the ISB-S CD4 dataset grouped by disease condition. 677 TCR specificity clusters were found in common across different severities of COVID-19. h Venn diagram showing overlap between consensus COVID-19-associated T cell clusters (taken from intersection of disease conditions in Fig. 1G) and healthy donors for repertoires in the ISB-S CD4 dataset. Among the 677 T cell clusters commonly found across all levels of COVID-19 severity, 474 clusters were exclusive to COVID-19 patients and not found within healthy donors.
Recent sequence similarity approaches have been developed to determine TCR specificity clusters for motif-based prediction of antigen specificity and identification of key conserved residues that drive TCR recognition27,28,29. We used the Grouping of Lymphocyte Interactions by Paratope Hotspots version 2 (GLIPH2) algorithm29 to cluster the TCR sequences based on predicted antigen specificity for motifs associated with different disease conditions in the ISB-S datasets. We also used the Optimized Likelihood estimate of immunoGlobulin Amino-acid sequences (OLGA) algorithm30 to calculate the generation probability (pGen) of the clonotypes contained in the clusters identified from the GLIPH2 analysis. Low pGen clonotypes are considered private and not shared widely in the population, while high pGen clonotypes are considered public and shared in a large proportion of the population due to convergent recombination22,31. We found that the mild and moderate disease conditions had both relatively lower pGen scores and higher median frequency clusters compared to the severe disease and healthy donor conditions for both the ISB-S CD4 and CD8 datasets (Fig. 2E, S4G, Supplementary Dataset S8–S10). Visualization of individual clusters revealed that the mild and moderate disease conditions had clonotypes with the highest proportional representation, including motifs AGQGA%E, S%AAG, SL%AG, and SLQGA%YE (the % character corresponds to a wildcard amino acid) for the ISB-S CD4 dataset (Fig. 2F) and motifs SEG%NTDT, SLDSGGA%E, SL%SGGANE, SLAA% for the ISB-S CD8 dataset (Fig. S4H). Using set analysis within the ISB-S CD4 dataset, we found that the motif-based clustering of T cells via GLIPH2 algorithm successfully identified 677 CD4 T cell clusters that are common across all levels of severity of COVID-19 (Fig. 2G). Among these 677 CD4 T cell clusters, 474 were exclusive to COVID-19 and not found in healthy donors (Fig. 2H). Similarly, for the ISB-S CD8 dataset, we found 51 consensus clusters, 35 of which were exclusively found in COVID-19 (Fig. S4I, J). We provide here all identified clusters and motifs with associated CDR3 sequences, V gene usage, and J gene usages, along with clonotype pGen scores and the identified COVID-19-associated clusters.
Transcriptional signatures of clonal expansion and associations with disease severity
In order to investigate the relationship between the enriched clonotypes and their transcriptomes, we performed dimensionality reduction on 137,075 CD4 T cell single-cell RNA sequencing samples that had CDR3 sequences associated with identified GLIPH2 clusters. The transcriptomes were projected to a two-dimensional space by uniform manifold approximation and projection (UMAP) (Fig. S5A). Clustering was performed using the Louvain algorithm, revealing 12 clusters with differentially expressed gene signatures (Fig. 3A, S5C). Overall, a stark contrast was observed in the clustering patterns of cells from healthy donors and COVID-19 patients in the UMAP, where cells from healthy donors were concentrated in clusters 3 and 4, while the cells from COVID patients were mainly found in cluster 6. Cluster 6 contained the proliferating subset of T cells with high degrees of clonality (Fig. 3A, S5B), suggesting phenotypic correlates of clonal expansion. Moreover, a high density of cells in cluster 6 contained the top COVID-enriched TCR sequence motifs identified from GLIPH2 motif analysis, such as AGQGA%E, S%AAG, SL%AG, SLQGA%YE, S%SGTDT, SL%GTDT, SLS%TDT, and S%AGNQP (Figs. 2F, 3C). These clonally expanded cells containing COVID-enriched TCR sequence motifs highly expressed the gene GNLY, which encodes the cytotoxic granules of T cells, indicating that the cells in cluster 6 are primarily activated, proliferating cytotoxic T cells. We also found a correlation between clonotype expansion and COVID infection, with cells from COVID-19 patients exhibiting the highest density in effector phenotype-associated cluster 6, while healthy donor cells exhibited density in the naïve phenotype-associated clusters (Fig. 3B). We also found a higher association of lower pGen score, or private, clonotypes with cluster 6 compared to the high pGen score clonotypes (Fig. 3D, Supplementary Dataset S10), suggesting that these clones may be specific. However, a comparison of the proportion of cells for each disease condition in cluster 6 with healthy donors revealed cell proportion increases only for the moderate condition (Fig. 3E), despite increasing trends for all conditions. In contrast, the naïve cell subset in the UMAP plots indicated by the gene markers TCF7 and LEF1, were most abundant among healthy donors’ T cells in clusters 3 and 4, whereas few naïve T cells were observed in cluster 6 (Fig. 3B, S5D). Altogether, these results demonstrate relationships between clonal expansion, disease status, and cell phenotype, which can be extended to subsequence motifs.
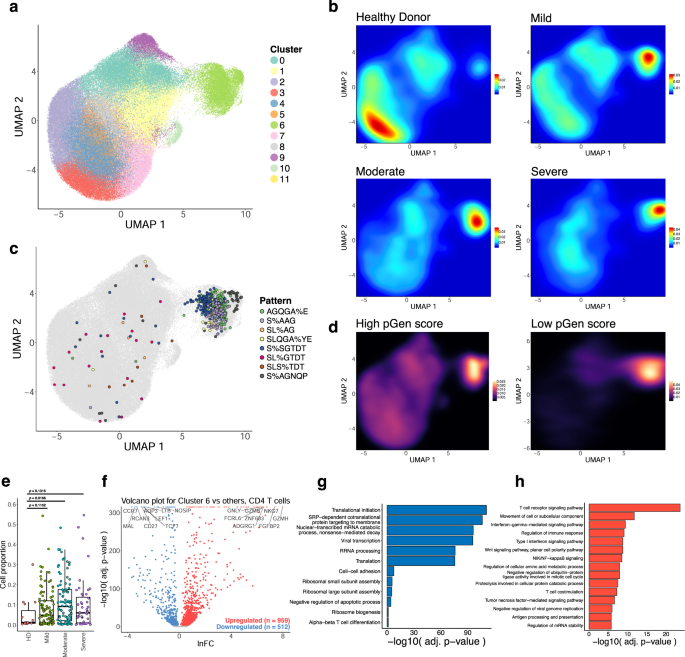
a UMAP visualization of 137,075 CD4 T cell single-cell transcriptomes from the ISB-S CD4 dataset pooled across samples and conditions. 12 clusters identified using the Louvain algorithm. b Two-dimensional density plot of cells from each disease condition (healthy donor, mild, moderate, severe) by UMAP coordinates. Red represents areas of high density of cells of a given condition; blue represents areas of low density. c UMAP visualization with cells labeled by top eight most frequent CD4 TCR clusters identified by the GLIPH2 analysis. d Two-dimensional density plot of cells with high or low pGen score clonotypes by UMAP coordinates. Yellow represents areas of high density of cells; black represents areas of low density. e Boxplots of clonally expanded cell proportions (in cluster 6) for each disease condition (cell count healthy donor = 544, mild = 3568, moderate = 5012, severe = 2336). Comparison between groups performed with two-sided Wilcoxon rank-sum test. f Volcano plot of differentially expressed genes between clonally expanded cells and all other cells in the ISB-S CD4 dataset (Cluster 6 cells = 5000, all other cells = 5000). Differential gene expression was performed with Seurat using the two-sided Wilcoxon rank-sum test; the Bonferroni corrected adjusted p-values and log fold-change of the average expression were used for visualization. g Bar plot of biological processes (BP) pathway terms associated with downregulated genes (clonally expanded cells vs all other cells, q-value < 1e-4) by DAVID analysis. h Bar plot of biological processes (BP) pathway terms associated with upregulated genes (clonally expanded cells vs all other cells, q-value < 1e-4) by DAVID analysis.
We extended this analysis to the CD8 dataset to see if the associations between clonal expansion and disease severity are maintained. UMAP projection of 70,237 CD8 T cell single-cell transcriptomes and clustering revealed 15 clusters with differentially expressed gene signatures (Fig. 4A, S6A, S6C, Supplementary Dataset S11–S13). As with the CD4 dataset, we found clustering of cells with high degrees of clonality, distributed here across the clusters 0, 2, 3, 5, 7, 9, 10, 13, and 14 (grouped together as Expanded for further analysis) (Fig. 4A, S6B). We also found high density of top enriched GLIPH2 motifs in the Expanded group, including SEG%NTDT, SLDSGGA%E, SL%SGGANE, SLAA%, SQT%STDT, SP%SGSYE, SPGT%GYNE, and S%RQGAGGE (Fig. 4C, S4H). We observe a relatively higher density of cells from COVID-19 disease conditions in the Expanded group as compared to the healthy donors (Fig. 4B), with a low density of disease-associated cells in the non-Expanded clusters. Likewise, we found a more exclusive association between lower pGen score clonotypes and the Expanded group, particularly cluster 9 (Fig. 4D). A comparison of the proportion of cells for each disease condition in the Expanded group with healthy donors reveals cell proportion increases for all conditions (Fig. 4E). These results highlight the relationship between clonal expansion and disease severity, which is comparable to the results from the CD4 dataset.
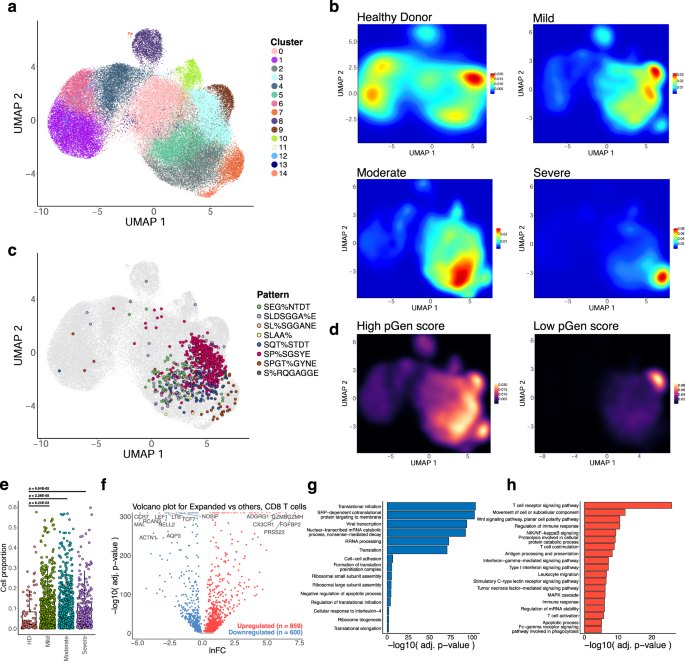
a UMAP visualization of 70,237 CD8 T cell single-cell transcriptomes from the ISB-S CD8 dataset pooled across samples and conditions. 15 clusters identified using the Louvain algorithm. b Two-dimensional density plot of cells from each disease condition (healthy donor, mild, moderate, severe) by UMAP coordinates. Red represents areas of high density of cells of a given condition; blue represents areas of low density. c UMAP visualization with cells labeled by top eight most frequent CD8 TCR clusters identified by the GLIPH2 analysis. d Two-dimensional density plot of cells with high or low pGen score clonotypes by UMAP coordinates. Yellow represents areas of high density of cells; black represents areas of low density. e Boxplots of clonally expanded cell proportions (in Expanded group) for each disease condition (cell count healthy donor = 2579, mild = 18,622, moderate = 15,743, severe = 7159). Comparison between groups performed with two-sided Wilcoxon rank-sum test. f Volcano plot of differentially expressed genes between clonally expanded cells and all other cells in the ISB-S CD8 dataset (Expanded group cells = 5000, all other cells = 5000). Differential gene expression was performed with Seurat using the two-sided Wilcoxon rank-sum test; the Bonferroni corrected adjusted p-values and log fold-change of the average expression were used for visualization. g Bar plot of biological processes (BP) pathway terms associated with downregulated genes (clonally expanded cells vs all other cells, q-value < 1e-4) by DAVID analysis. h Bar plot of biological processes (BP) pathway terms associated with upregulated genes (clonally expanded cells vs all other cells, q-value < 1e-4) by DAVID analysis.
To investigate the gene expression changes that occur with clonal expansion, we performed differential expression (DEX) analysis between cluster 6 cells versus all other cells for the CD4 dataset (Fig. 3F) and the Expanded group cells versus all other cells for the CD8 dataset (Fig. 4F, Supplementary Dataset S14, S15). Using a threshold of q-value < 1e-4, we found 512 downregulated genes and 959 upregulated genes for the CD4 T cell DEX, as well as 600 downregulated genes and 859 upregulated genes for the CD8 T cell DEX. Volcano plots for both T cell types revealed upregulation of cytotoxicity-associated transcripts such as granzymes and granulysin and downregulation of naïve phenotype associated markers such as TCF7 and LEF1. Comparison of UMAPs of the individual subpopulation phenotype markers also showed a correlation between cluster 6 or the Expanded group clusters and effector-related markers such as GZMA, PRF1, NKG7, and GNLY, with downregulation of naïve-related markers such as TCF7 and LEF1 (Figs. S5D, S6D). Functional gene annotation analysis with DAVID32 revealed enriched pathways terms such as TCR signaling pathway, regulation of immune response, NF-kB signaling, IFN-gamma mediated signaling, and TNF-mediated signaling pathways were upregulated in clonally expanded clusters (Figs. 3H, 4H, Supplementary Dataset S16) while terms such as translational initiation, viral transcription, translation, and ribosomal subunit assembly were downregulated (Figs. 3G, 4G) for both CD4 and CD8 differential expression analyses. Therefore, we find that clonally expanded CDR3 sequences and motifs are highly associated with effector T cell phenotypes at both the individual gene and functional pathway levels while downregulating a number of mRNA processing-related programs.
Machine learning models for disease severity
To determine whether the constitutive sequence motifs in the CDR3 sequence of the TCR contain sufficient information to be predictive of COVID-19 infection, we trained several classical supervised machine learning (ML) algorithms on the repertoires from the ISB-S CD4 and ISB-S CD8 datasets. We implemented Random Forests (RF), Support Vector Machines (SVM), Bernoulli Naïve Bayes (BNB), Gradient Boosting Classifiers (GBC), and K-Nearest Neighbors (KNN) on frequency matrices of overlapping 3-mer or 6-mer amino acids adapted from the TCR repertoires. ML models were trained as binary classification tasks to predict mild, moderate, or severe COVID-19 TCR repertoires from healthy donor repertoires for either CD4 or CD8 ISB-S datasets. A total of 12 models were trained, with the permutations varying in (1) classifying different levels of COVID severity (HD vs Mild, HD vs Moderate, HD vs Severe), (2) CD4 vs CD8 T cell receptors, and (3) 3mer vs 6mer representation of the TCR data. Training and testing sets were partitioned with an 80:20 ratio, then for 500 iterations, the algorithms were trained on a random 80% of the training set and evaluated for performance on the test set. We found that RFs, GBCs, and SVMs generally had strong classification performance across the board, compared to KNNs or BNB. Particularly strong classification performance was observed in the models trained on the CD8 T cell dataset’s 6mer representation, with certain predictors approaching near-perfect scores (AUROCs = 0.93–0.99) (Fig. 5A, S7A, Supplementary Dataset S17). Notably, the ML models had a higher performance for classifying moderate COVID repertoires from HD than for classifying severe COVID repertoires from HD. This is consistent with the increased separation of the moderate repertoires observed in the PCA analysis. Model performance in classifying HD from COVID repertoires was much stronger overall in the CD8 T cell subset compared to the CD4 T cell subset, suggesting that immune signatures of COVID infection are much more salient in CD8 T cells’ repertoires compared to CD4 T cells’ repertoires (Fig. 5A, Fig. S7A). Overall, these results demonstrate that ML-based methods can identify with high classification accuracy samples from COVID-19 patients of varying severity based on CDR3 sequences features, particularly for moderate disease conditions; however, it should be noted that the performance of these methods have only been demonstrated using the ISB-S datasets and may not be generalizable to other TCR repertoire datasets or for COVID-19 patients more broadly.
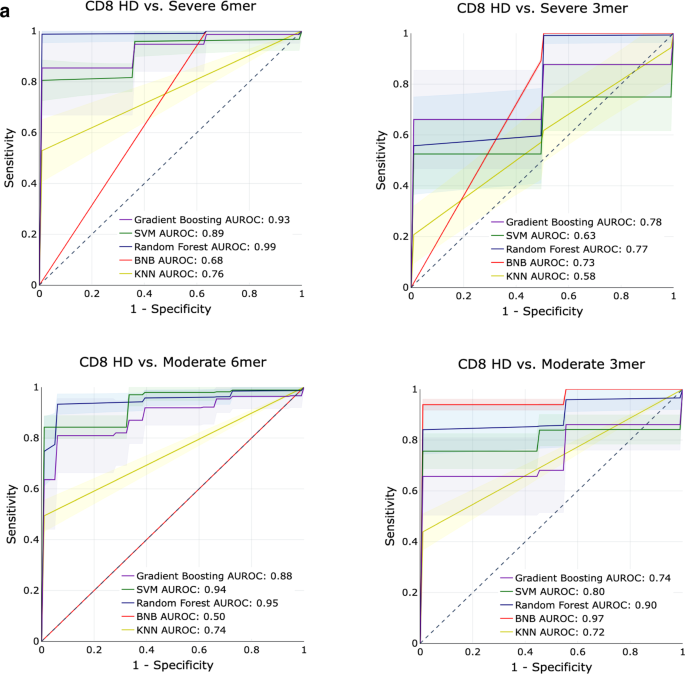
a AUROC curves for five machine learning models (gradient boosting trees, support vector machines, random forests, Bernoulli Naïve Bayes, and k-nearest neighbors) using 6-mer (left) and 3-mer (right) representations of TCR repertoire data. Models were trained to predict disease severity (moderate, severe) vs healthy donors for CD8 samples. Training and evaluation were performed using 500 iterations per model, average performance +/− 1 standard deviation shown on individual plots.